论文标题:Measuring social, environmental and health inequalities using deep learning and street imagery
期刊:Scientific Reports
作者:Esra Suel, John W. Polak, James E. Bennett, Majid Ezzati
发表时间:2019/04/18
数字识别码: 10.1038/s41598-019-42036-w
原文链接:http://t.cn/EXDZXWb
微信链接:https://mp.weixin.qq.com/s/JG3xn9WcVrQV1CZbdodNpQ
根据《科学报告》本周发表的一项研究 Measuring social, environmental and health inequalities using deep learning and street imagery,一种能分析公开街道图像数据的深度学习计算机方法,可以用于检测英国城市社会、经济、环境和健康方面的不平等情况。
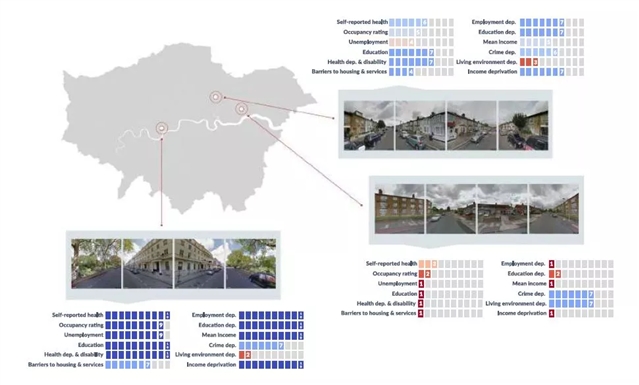
图1:这项分析使用了街道图像和结果(标签)数据。图源:Esra Suel等
伦敦等大城市存在大量不平等现象,而详细的数据测量对于制定、评估那些减少不平等的政策来说非常重要。但是只有少数国家拥有能进行实时测量、链接全面的统计数据集。
英国帝国理工学院的Esra Suel和同事利用深度学习方法训练了一种计算机程序,该程序通过可公开获取的街道图像和政府统计数据,能检测英国四大主要城市(伦敦、伯明翰、曼彻斯特和利兹)的不平等情况。经过525860张伦敦城市图像(对应156581个邮政编码)的训练后,再用1%的西米德兰兹郡、大曼彻斯特郡和西约克郡图像进行微调,该程序对另外三座城市预测结果的准确度就能与伦敦不相上下。
作者假设城市和城市生活的一些特征——如住房质量和生活环境——拥有计算机可识别的直接视觉信号,这些信号包括建筑材料和失修情况、汽车或当地商铺。结合政府对该城市住房条件、平均收入或死亡率和发病率等结果的统计数据,这些图像或能用来训练一种计算机程序,以检测没有统计数据的城市中的不平等情况。
摘要:Cities are home to an increasing majority of the world’s population. Currently, it is difficult to track social, economic, environmental and health outcomes in cities with high spatial and temporal resolution, needed to evaluate policies regarding urban inequalities. We applied a deep learning approach to street images for measuring spatial distributions of income, education, unemployment, housing, living environment, health and crime. Our model predicts different outcomes directly from raw images without extracting intermediate user-defined features. To evaluate the performance of the approach, we first trained neural networks on a subset of images from London using ground truth data at high spatial resolution from official statistics. We then compared how trained networks separated the best-off from worst-off deciles for different outcomes in images not used in training. The best performance was achieved for quality of the living environment and mean income. Allocation was least successful for crime and self-reported health (but not objectively measured health). We also evaluated how networks trained in London predict outcomes three other major cities in the UK: Birmingham, Manchester, and Leeds. The transferability analysis showed that networks trained in London, fine-tuned with only 1% of images in other cities, achieved performances similar to ones from trained on data from target cities themselves. Our findings demonstrate that street imagery has the potential complement traditional survey-based and administrative data sources for high-resolution urban surveillance to measure inequalities and monitor the impacts of policies that aim to address them.
阅读论文全文请访问:http://t.cn/EXDZXWb
期刊介绍:Scientific Reports (https://www.nature.com/srep/) is an online, open access journal from the publishers of Nature. We publish scientifically valid primary research from all areas of the natural and clinical sciences.
The 2017 journal metrics for Scientific Reports are as follows:
•2-year impact factor: 4.122
•5-year impact factor: 4.609
•Immediacy index: 0.576
•Eigenfactor® score: 0.71896
•Article Influence Score: 1.356
•2-year Median: 2
(来源:科学网)
特别声明:本文转载仅仅是出于传播信息的需要,并不意味着代表本网站观点或证实其内容的真实性;如其他媒体、网站或个人从本网站转载使用,须保留本网站注明的“来源”,并自负版权等法律责任;作者如果不希望被转载或者联系转载稿费等事宜,请与我们接洽。